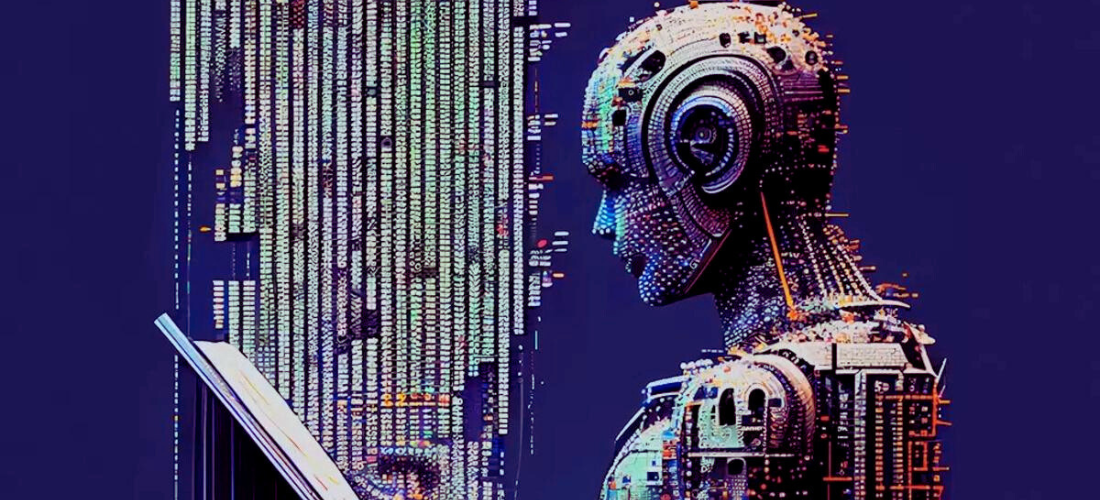
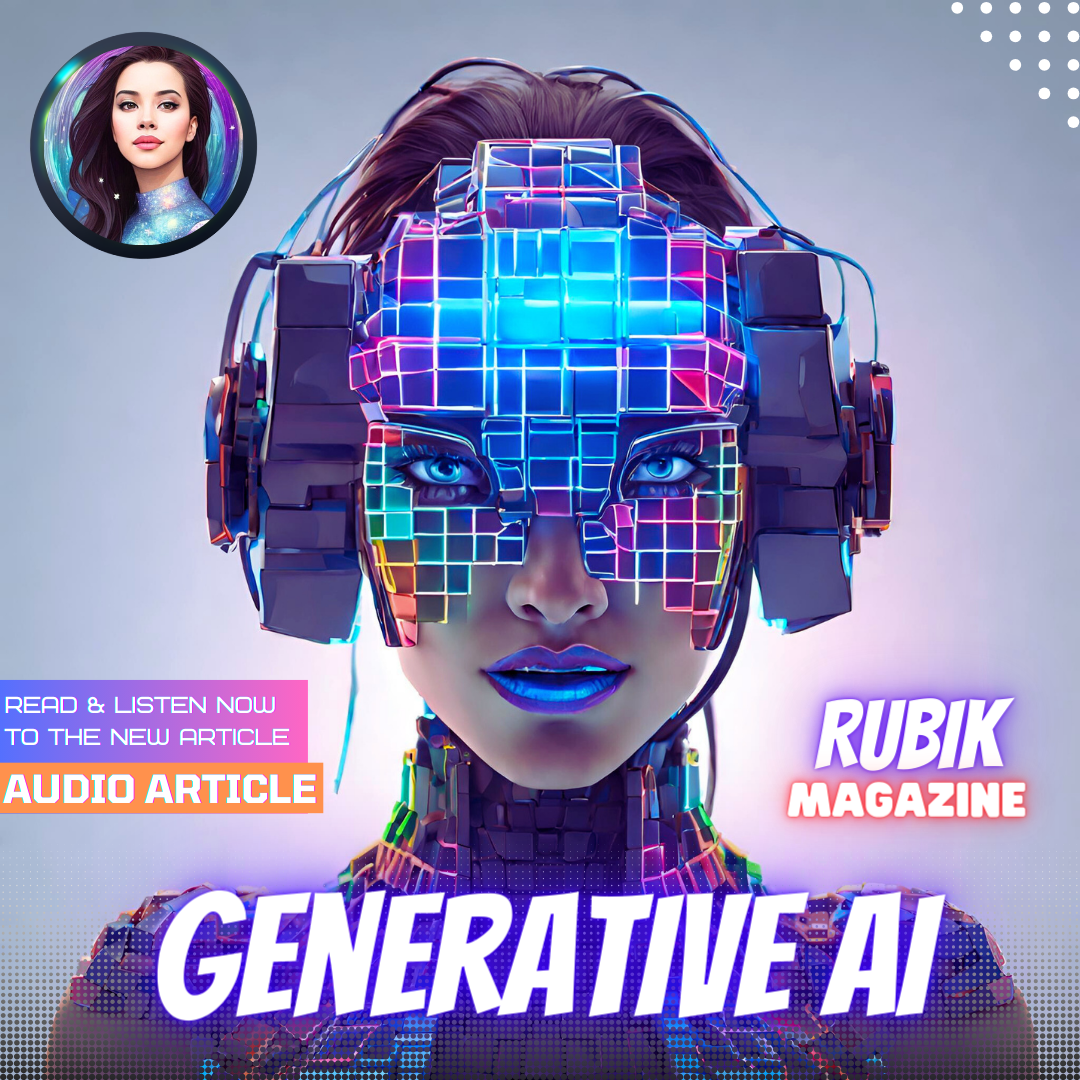
Introduction
Generative AI represents a branch of Artificial Intelligence (AI) focused on autonomous creation of data, content, and images. Its development has been driven by advances in deep learning algorithms, generative neural networks, and architectures such as Generative Adversarial Networks (GANs).
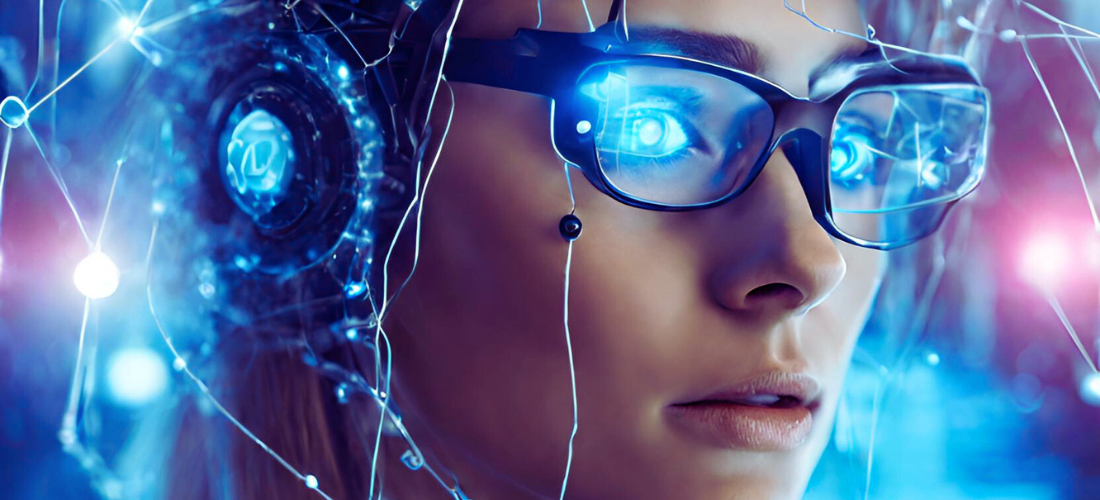
Key Architectures
1. Generative Adversarial Networks (GANs): GANs are a fundamental architecture in Generative AI. They consist of two neural networks, a generator, and a discriminator, competing to enhance data generation. The generator creates fake data, and the discriminator evaluates it. As they progress, the generator improves its ability to produce data indistinguishable from real data.
2. Transformers: Transformer-based neural networks have revolutionized natural language processing and text generation. Notable examples include GPT-3 and BERT, showcasing exceptional language understanding and generation capabilities.
3. Variational Autoencoders (VAEs): VAEs are another significant technique in data generation. They are ideal for modeling high-dimensional data and have been applied in fields like image generation.
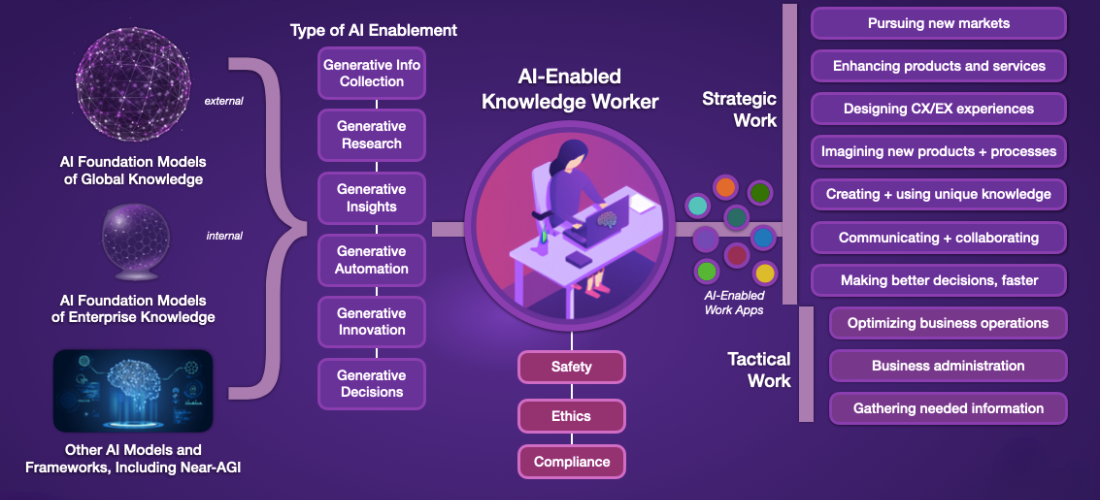
Generative Machines
1. Text Generation: GPT-4: The fourth iteration of the GPT series has taken text generation to a new level. With remarkable natural language capabilities, GPT-4 can produce coherent and persuasive content in various styles and topics.
2. Hyperrealistic Image Generation: GANs have advanced in creating images nearly indistinguishable from real photographs. This has applications in graphic content creation, video game design, and simulations.
3. Music and Artistic Creation: Generative AI has been employed to create music, paintings, and other forms of art. Projects like Aiva demonstrate AI's ability to generate creative works.
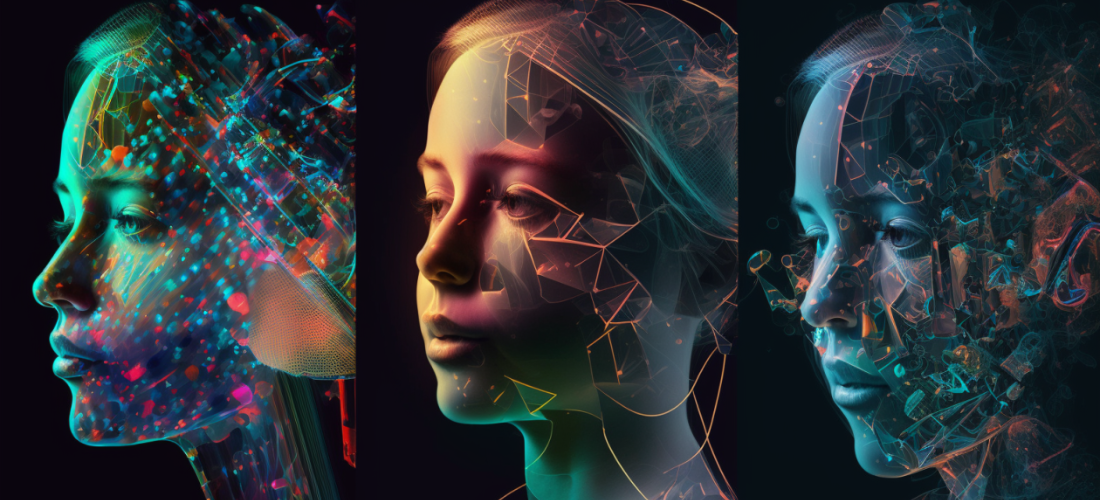
Applications and Use Cases
1. Automatic Translation and Multilingual Text Generation: Generative AI has revolutionized automatic translation, enabling precise content translation into multiple languages.
2. Product Design and Fashion: In the fashion and industrial design industry, GANs are used to generate prototypes and product styles.
3. Medicine and Drug Discovery: Generative AI is used in predicting molecular interactions and drug design.
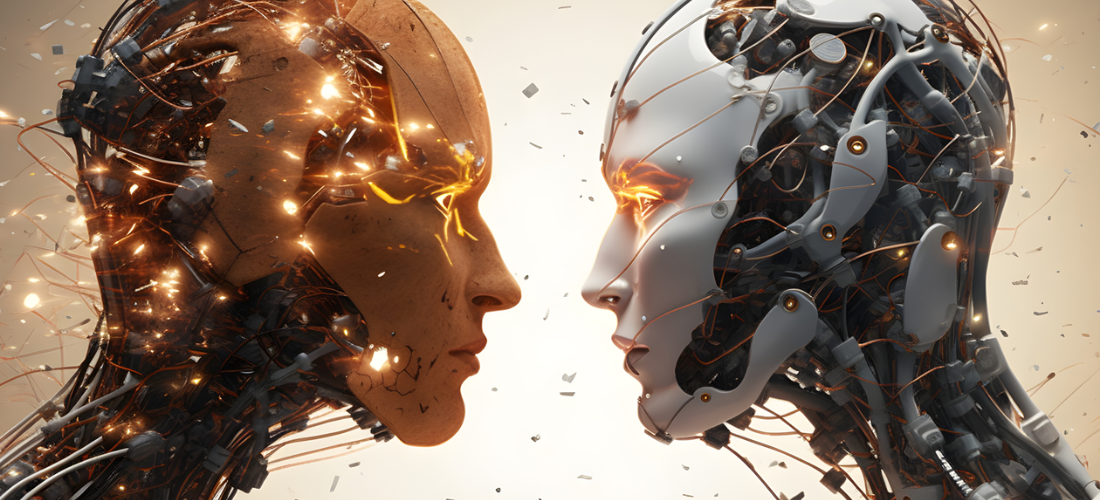
Challenges and the Future
Despite advancements, Generative AI faces significant challenges, including ethics in generating fake content, incomplete data interpretation and understanding, and the need for improved computational efficiency.
The future of Generative AI promises greater integration into society, with applications in education, entertainment, medicine, and more. Ethics and regulation will be crucial to guide its development.
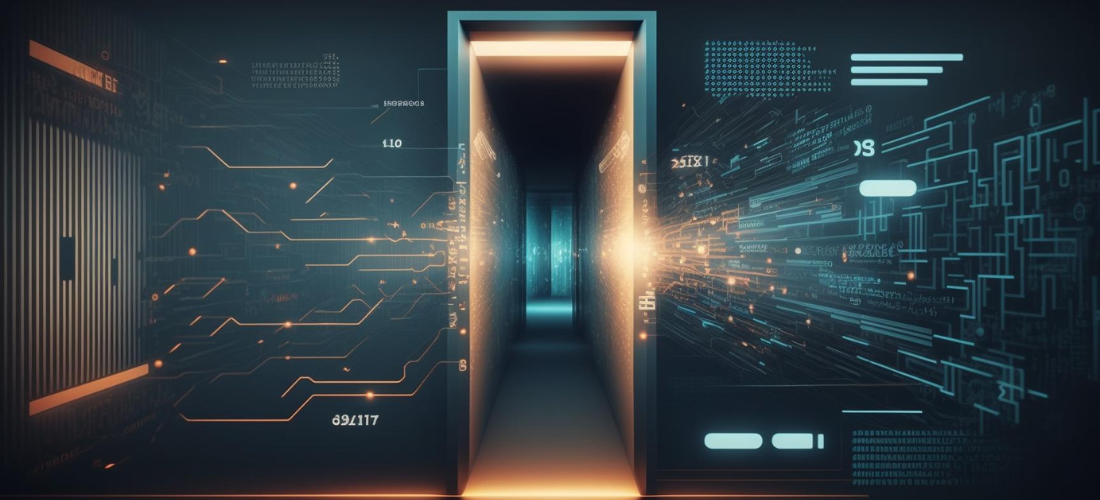
Conclusion
Generative Artificial Intelligence is continually evolving, and its applications are becoming increasingly astonishing. From content generation to product design and science, Generative AI has become a fundamental pillar in computer engineering, and its influence will only continue to grow in the coming years.
This article has provided an in-depth and technical insight into Generative AI, highlighting key concepts, recent advancements, innovative applications, and future challenges. The world of Generative AI is exciting and ever-evolving, and it will be fascinating to see where it leads us in the future.
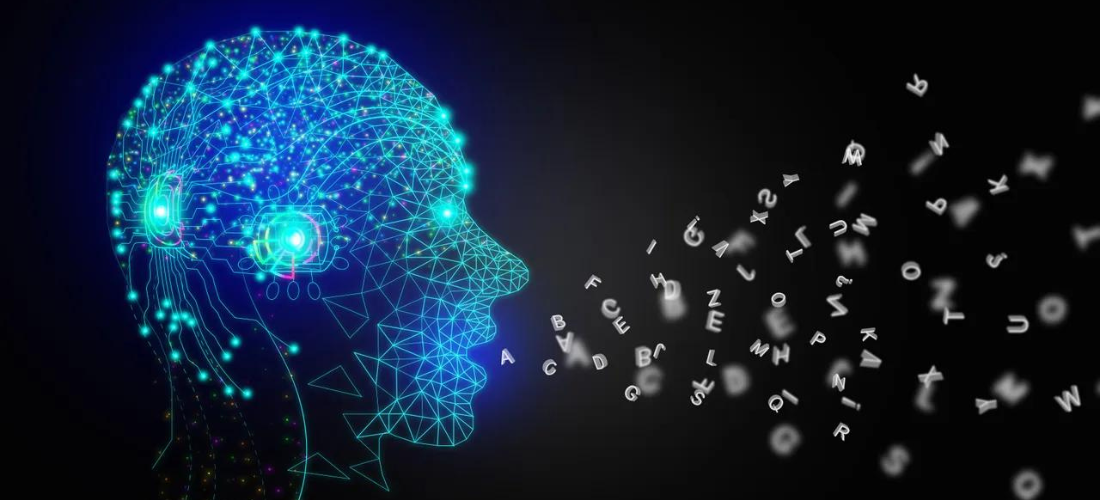