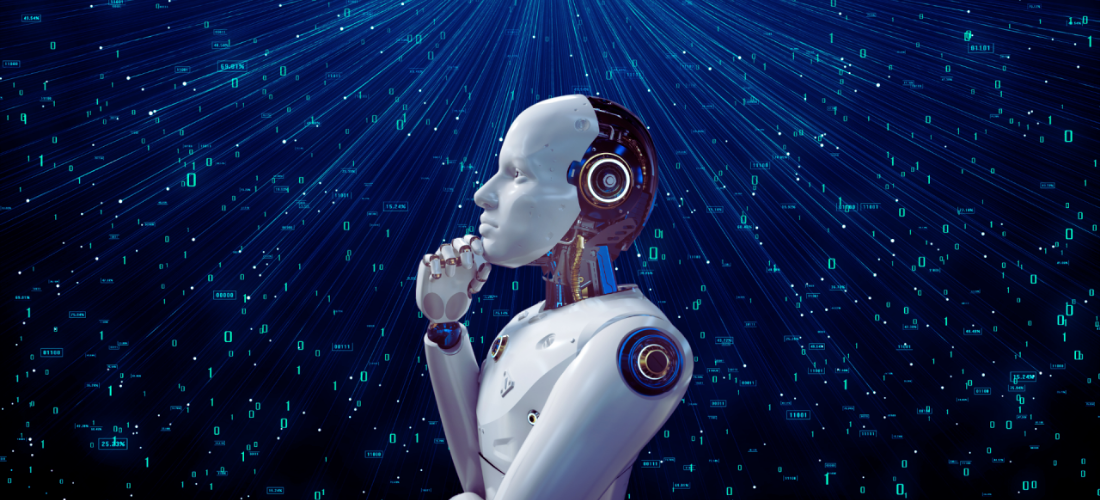
Algorithms Without Barriers: How to Combat Bias and Create Fair and Responsible Technologies
In the current environment, where artificial intelligence (AI) and machine learning are technological cornerstones, algorithms play a crucial role in a variety of processes, from candidate selection for jobs to credit approval. However, when algorithms exhibit bias, they can reinforce social inequalities and generate discriminatory outcomes. This type of bias is not only a technical challenge but also an ethical one, requiring developers to act responsibly and with awareness.
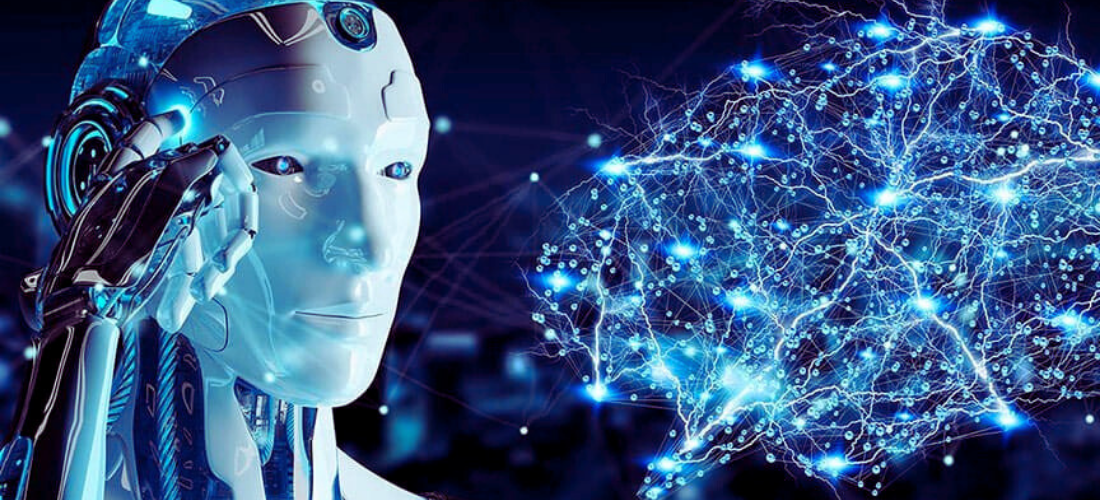
What is Algorithmic Bias?
Algorithmic bias occurs when an algorithm unfairly favors or discriminates against certain groups. It can be the result of biased training data, incorrect model design decisions, or a lack of diversity in development teams.
Sources of Algorithmic Bias
- Biased Data: If the data used to train algorithms reflect historical prejudices, these biases are replicated in the outcomes.
- Model Design: Decisions made during the model creation process, such as variable selection or focusing on certain metrics, can also introduce biases.
- Lack of Diversity: Homogeneous development teams may overlook how an algorithm disproportionately affects different groups of people.
Examples of Algorithmic Bias
- Automated Recruitment: Some algorithms have excluded female candidates in the selection process due to biases in historical data.
- Facial Recognition: Facial recognition systems have shown higher error rates in identifying individuals with darker skin tones.
- Credit Models: Some algorithms assign lower scores to marginalized communities, perpetuating past biases.
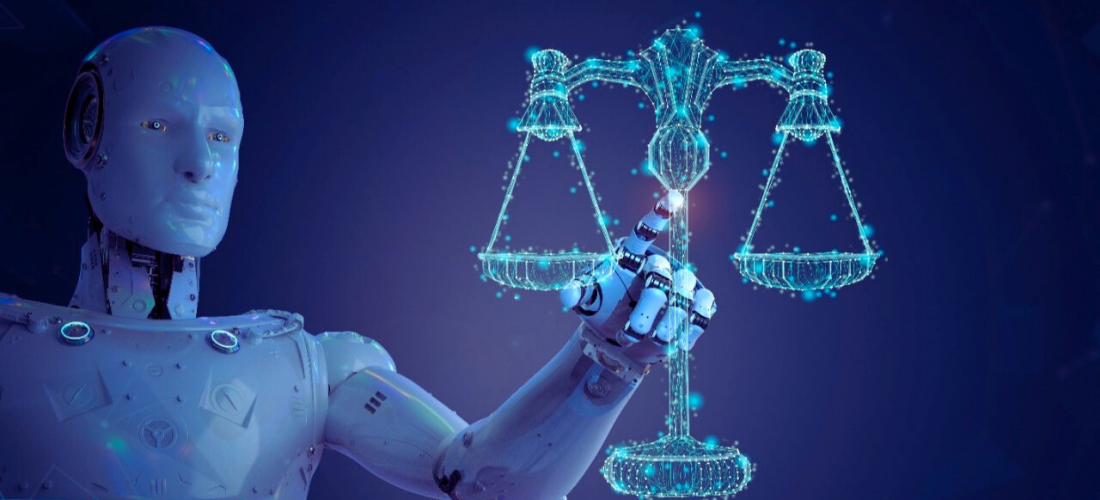
How to Address Bias in Algorithm Development
- Ethical Design: It is crucial to integrate equity and transparency principles from the outset of the project, establishing clear ethical guidelines.
- Data and Model Audits: Before training a model, detailed audits are necessary to identify potential biases in the data and the model.
- Improving Training Data: Using more representative data that includes diverse populations is essential to reduce historical biases.
- Transparency and Explainability: Systems should be transparent, allowing users to understand how decisions are made.
- Diversity in the Team: Diverse development teams help identify biases and develop more inclusive solutions.
- Interdisciplinary Collaboration: Incorporating experts in ethics and sociology can enrich the algorithm design and prevent undetected biases.
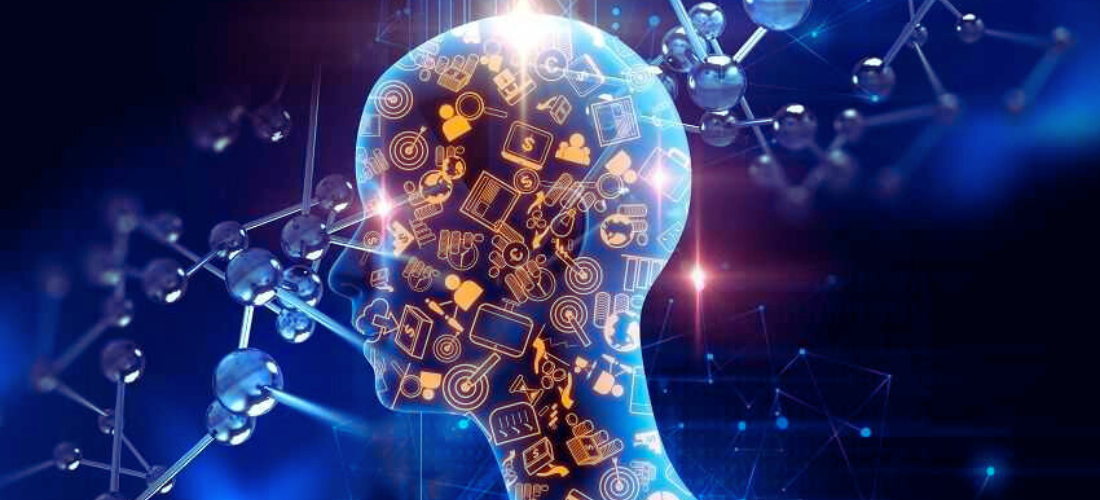
Mitigating Bias Through Regularization and Adjustments
Regularization techniques, such as penalizing biases in the cost function or adversarial training, can help create fairer models. These methods allow algorithms to predict without relying on sensitive factors such as gender or race.
Continuous Evaluation Post-Deployment
Once models are in production, it is essential to monitor their performance and conduct periodic audits to identify and correct unexpected biases. User feedback and constant analysis are crucial to ensure that systems do not introduce new inequalities.
The Future of Responsible Development
Developers have the responsibility to create technologies that are not only efficient but also fair and responsible. Actively addressing biases not only enhances trust in technologies but also contributes to a more inclusive and equitable future for all.
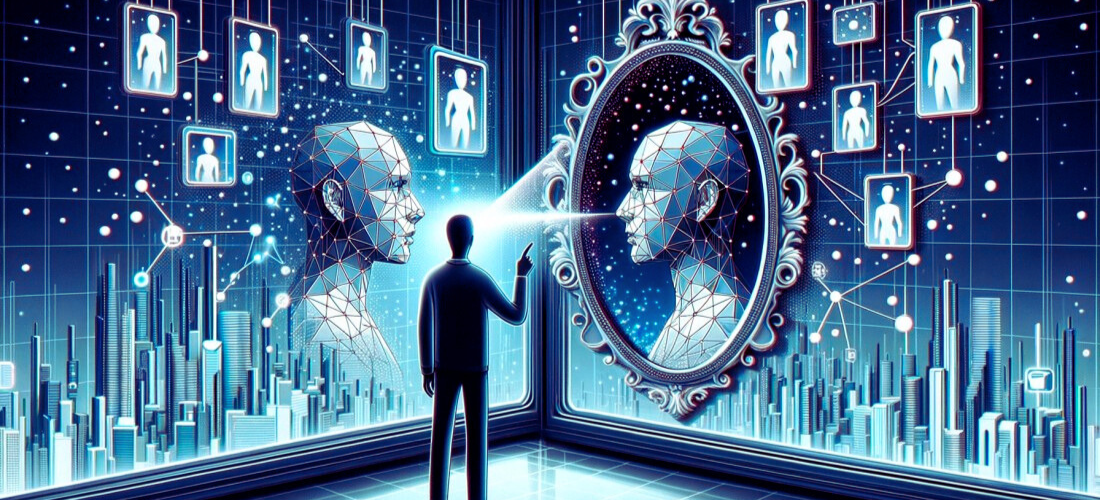
The Role of Regulation in the Fight Against Algorithmic Bias
The impact of biased algorithms not only affects individuals but also has a detrimental effect on society as a whole. Therefore, governments and international organizations are increasingly taking steps to regulate the use of artificial intelligence and require the implementation of ethical standards. Laws such as the General Data Protection Regulation (GDPR) in Europe and the EU's AI Act require organizations to implement practices that ensure non-discrimination in their automated systems.
Regulation should not only focus on preventing biases but also on ensuring that developers and companies are held accountable for the impact of their technologies on society. Creating legal frameworks that require companies to audit their systems, report failures, and take corrective actions could be a key tool in minimizing the risks of algorithmic bias.
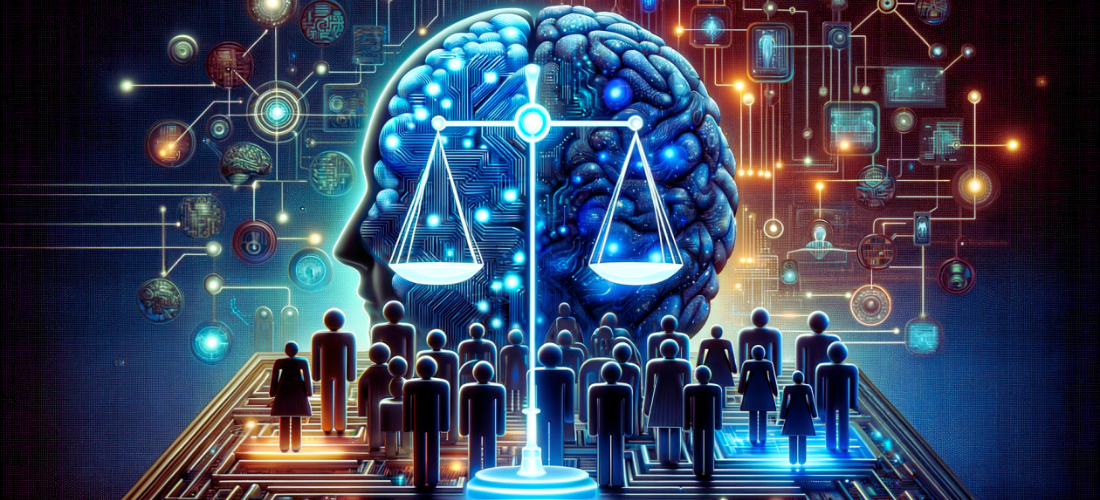
The Impact of Education and Awareness on AI Ethics
In addition to regulation, it is crucial to foster greater awareness of ethics in artificial intelligence within the tech community. Education in technological ethics should be an integral part of the training for developers, engineers, and AI designers. By teaching them to identify biases in data, models, and the decisions they make, the risks of creating unjust systems can be significantly reduced.
Universities, research centers, and tech companies should collaborate to incorporate ethical principles into AI education. This not only prepares the next generation of developers to face ethical challenges but also encourages companies to make more responsible and transparent decisions, knowing that ethics are deeply integrated into the development process.
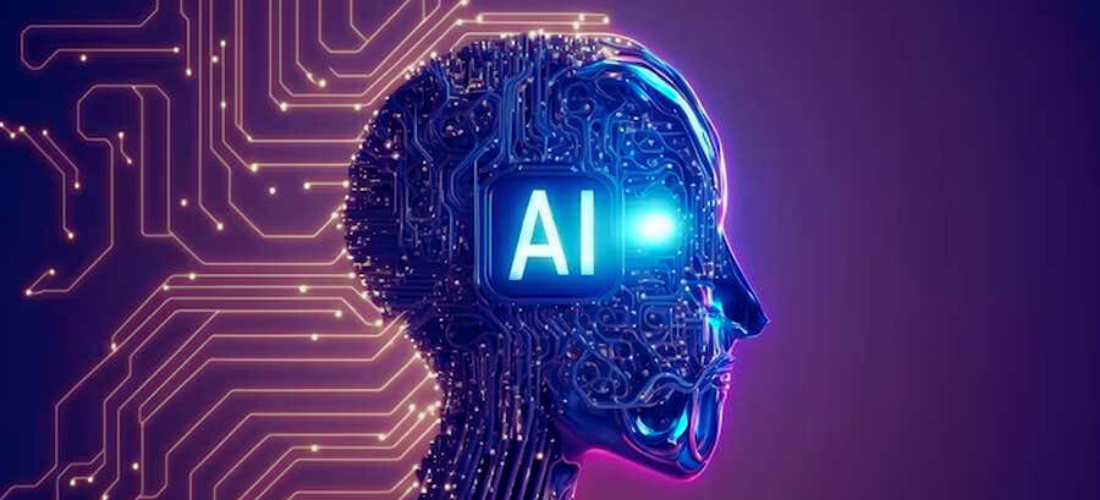
Conclusion
Creating responsible and unbiased technologies is an effort that involves everyone: developers, companies, regulators, and educators. Combating algorithmic bias requires concerted action, including data audits, inclusive design, strict regulations, and greater ethical awareness in the AI field. Only through these efforts can we build systems that are not only technically efficient but also fair, equitable, and inclusive for all individuals, regardless of their background or characteristics.